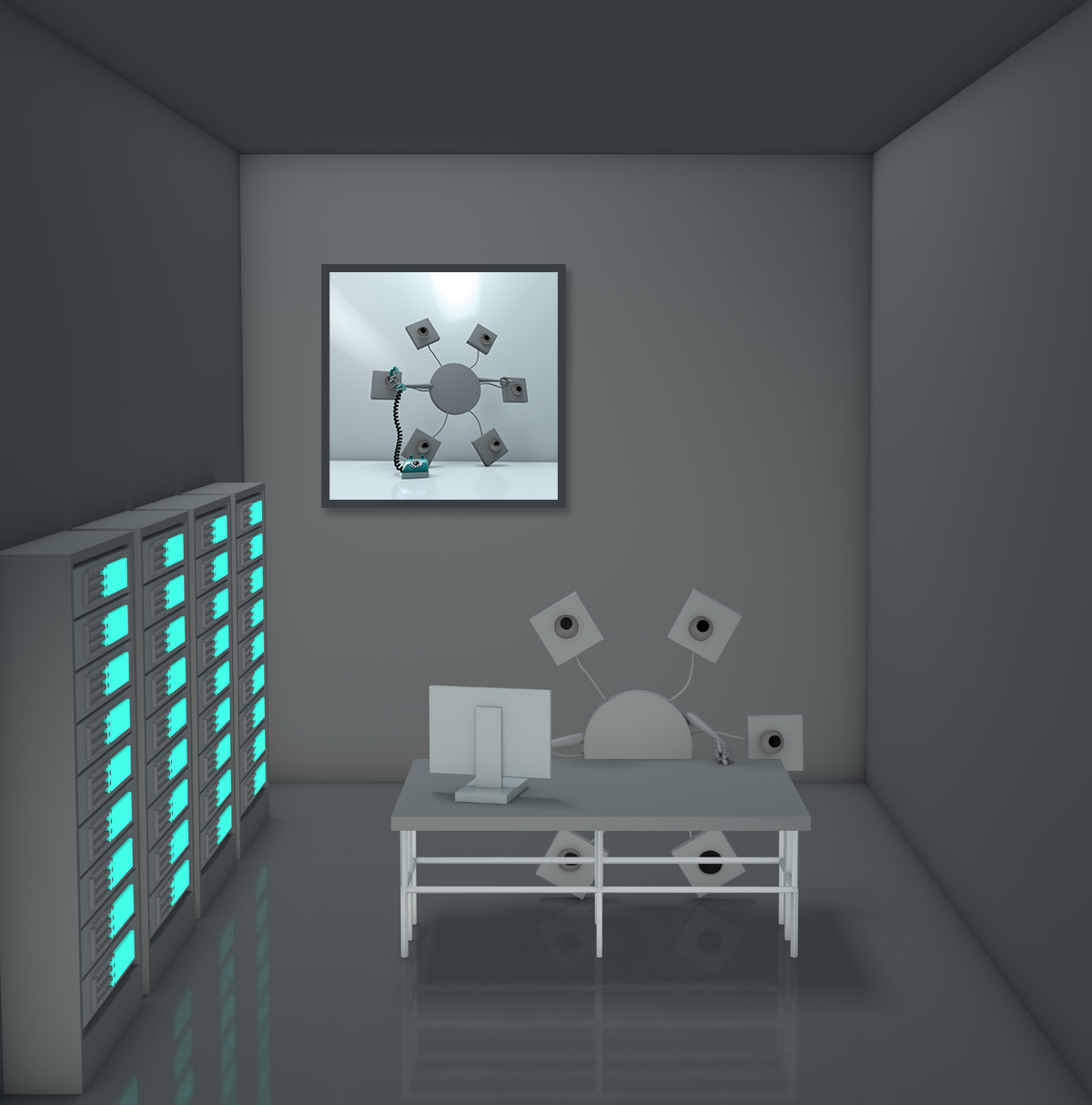
DATA ANALYSIS, INTEGRATION & STEWARDSHIP
Recently, we have seen an explosion of -omics data. Many analysis pipelines for genomics, transcriptomics, proteomics and metabolomics are available, but to make integrated use of those data and to translate them into actionable knowledge (e.g. new bioengineering strategies, new diagnostic routines, new drug targets) is a major bottleneck.
This is particularly true for researchers, who do not have access to state-of-the-art bioinformatics expertise, tools or analysis environments. The X-omics infrastructure will enable efficient data integration for data generated within X-omics and external datasets. X-omics adopts the FAIR principles and takes them a step further by helping researchers to: make data FAIR at the source, facilitate reuse of their data and make their data analysis workflows transparent and reproducible.
To facilitate analysis, integration & stewardship of X-omics data, X-omics will start developing:
An integrated strategy for experimental design that will enable the analysis of complex samples with multiple omics technologies.
A data infrastructure based on FAIR principles and design methods to combine different data sources from analytical omics-platforms and other related data to obtain a comprehensive view of complex systems.
Development of more sensitive enrichment technologies to improve depth of analysis of post-translational protein modifications.
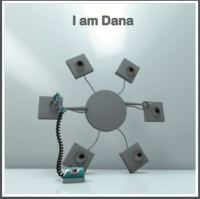
The main area of expertise of the data facilities, that are part of the X-omics research infrastructure, consists of integration of X-omics data, FAIRification of -omics data, reproducible data analysis and workflow management. The services are described below.
Upstream data analysis
- Diagnostics quality variant calling
- Long read analysis and optical mapping
- Differential expression analysis
- Quantitative, LC-MS & phospho-proteomics analysis
- Quantitative Metabolomics analysis
- UntargetedMetabolomicsanalysis
- Methylation analysis
- Microbiomics analysis
- Lipidomics analysis
Downstream data analysis
- Somatic variant analysis and structural variations
- RNA Isoform & splicing analysis
- Pathway analysis for RNA-Seq, Methylation and Untargeted Metabolomics
- Next-generation metabolic screening
- Metabolite set enrichment analysis
- Genotype linkage to phenotype and rare diseases
- FAIRification, Data Ontology support and Text mining
Multi-omics data analysis & integration
- Proteogenomics combining Proteomics with DNA, RNA or Long reads
- Pathway and gene network integration
- Statistical integration of multi-omics data
All services mentioned above are custom services.
Please contact our helpdesk for a quote with turnaround times and prices based on your research question.
FAIR Data Cube
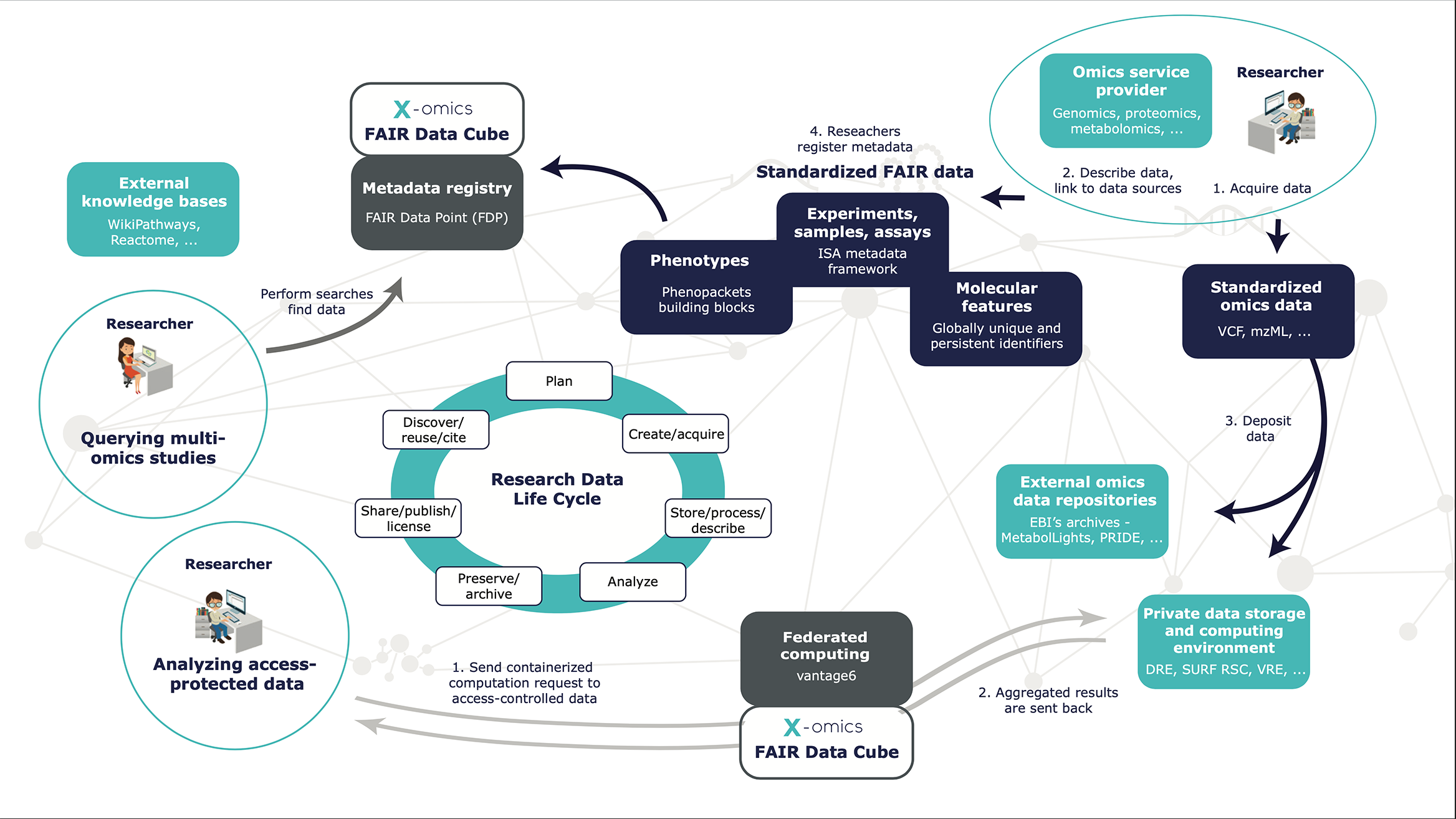
The FAIR Data Cube (FDCube) is an important infrastructure component in the Netherlands X-omics initiative. It is a set of tools and services that helps researchers in distinct stages of the Research Data Life Cycle with:
- Creating rich and machine readable metadata for single and multi-omics datasets;
- Making -omics data findable and accessible for reuse;
- Facilitating analysis and integration of -omics data from different sources.
Creation of multi-omics data and metadata
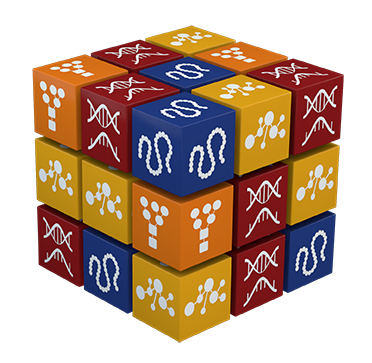
Querying multi-omics studies
Multi-omics analysis
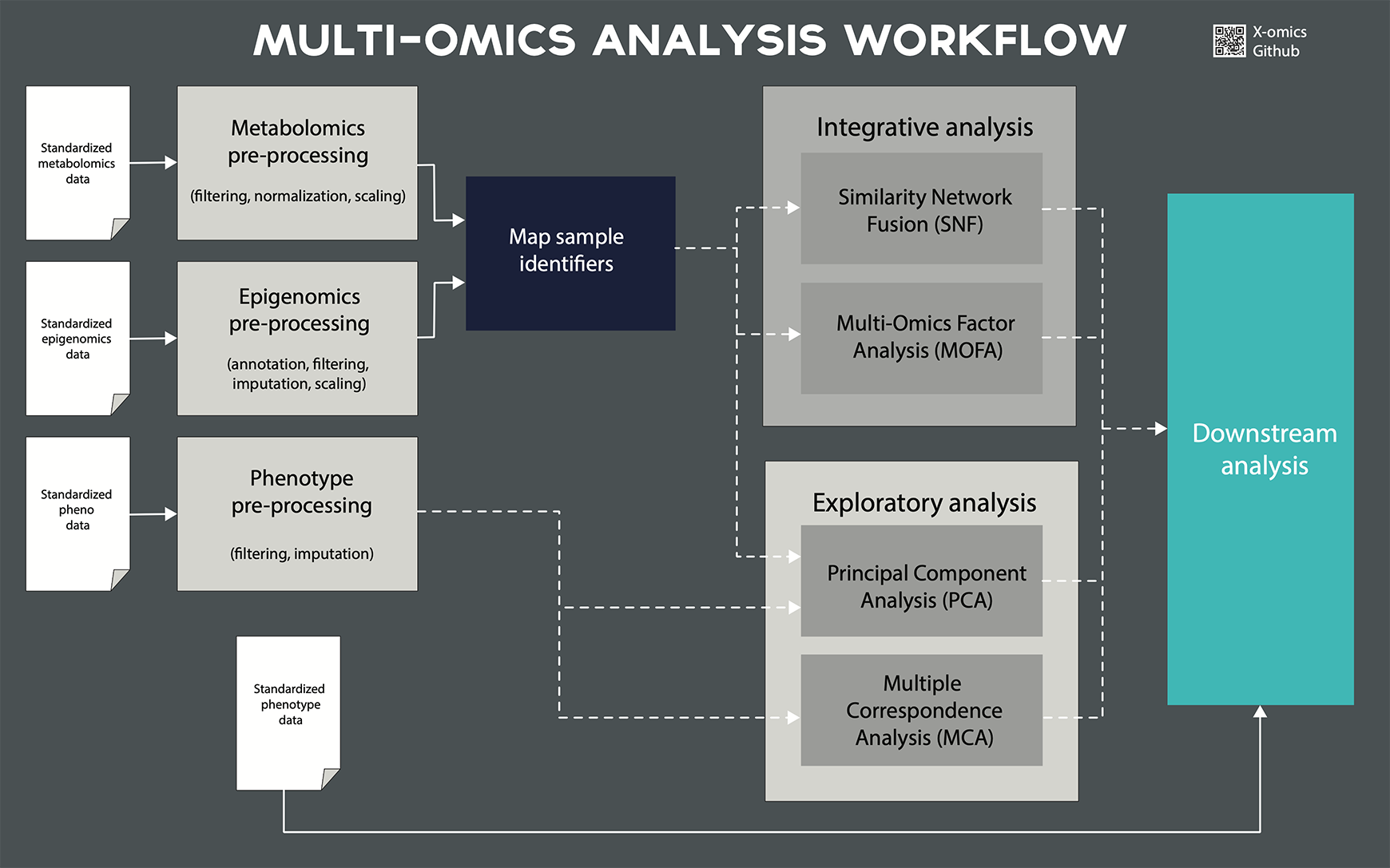
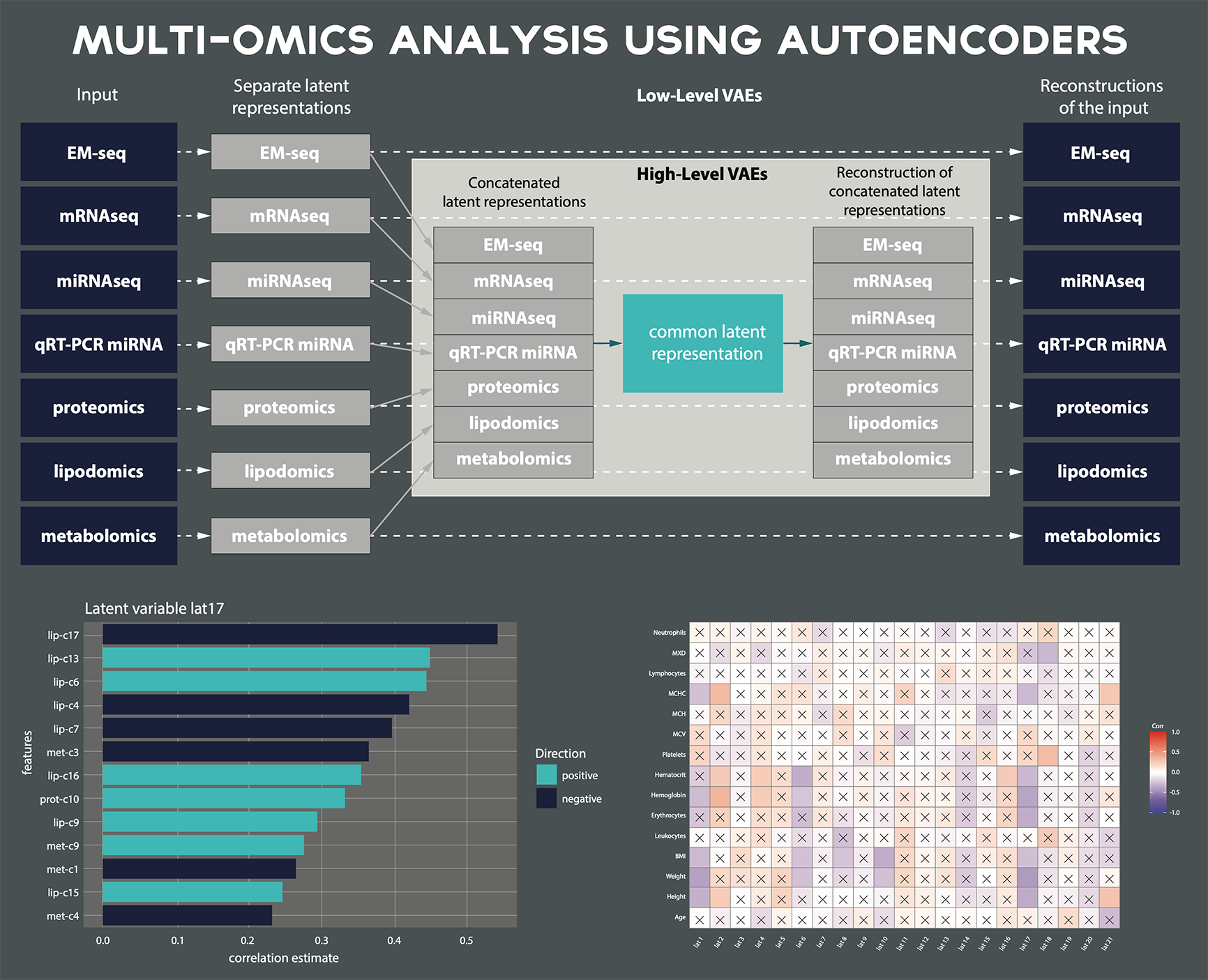